サマリー:
- Darktrace/OT leverages machine learning to provide actionable preventative analytics, relevant real time anomaly based threat detection, and a variety of response capabilities as a full suite protection for OT/ICS operations Purdue levels 5-0.
- Self-Learning AI detects and responds to cyber threats including malicious or non malicious insiders and supply chain attacks.
- Darktrace/OT deploys passively within NERC CIP environments providing visibility without the need for any external connectivity or threat intelligence updates.
What is FERC?
The US Federal Energy Regulatory Commission (FERC) is responsible for the regulation of the wholesale electricity and natural gas transmission. FERC sits above the North American Electric Reliability Corporation (NERC) which is responsible for the development and enforcement of reliability standards for the US bulk power system. NERC CIP reliability standards are standards enforced by NERC to ensure the safety and protection of the bulk electric system.
What is FERC order 887?
In review of the CIP requirements, FERC identified a security gap. The gap was that there is no requirement for internal network security monitoring (INSM) within the security perimeters of CIP networked systems. Without this requirement and protections in place, if an attacker was to breach the security perimeter of the CIP networked environment, the victim organization would have no capability of detecting and alerting to what the adversary is doing within the security perimeter.
FERC Order 887 is a final rule issued intended to direct NERC to develop new or modified reliability standards requiring internal network security monitoring INSM within Critical Infrastructure Protection (CIP) networked environments. A focus is placed on anomaly based detection used within the security perimeter so that threats without known rules and signatures associated, including insider threat and supply chain attacks, can be detected based on anomalous network activity within the CIP networked environment.
FERC order 887 specifically focuses on the need for addressing the INSM gap for BES high impact power generation systems with CIP networked environments with and without external connectivity and medium impact systems with external connectivity.
FERC Order 887 Requirements
1. Any new or modified CIP Reliability Standards should address the need for responsible entities to develop baselines of their network traffic inside their CIP-networked environment for BES Medium impact with external routable network connectivity and high impact with or without external routable network connectivity.
2. Any new or modified CIP Reliability Standards should address the need for responsible entities to monitor for and detect unauthorized activity, connections, devices, and software inside the CIP-networked environment. This should be done so that sophisticated threats including those that may already have persistent access to CIP networked systems, insider threats and supply chain threats can be detected at earlier stages.
3. Any new or modified CIP Reliability Standards should require responsible entities to identify anomalous activity to a high level of confidence by: (1) logging network traffic (we note that packet capture is one means of accomplishing this goal); (2) maintaining logs and other data collected regarding network traffic.
How does Darktrace support FERC order 887?
For security professionals to satisfy FERC order 887, it is ideal to deploy an INSM that leverages anomaly based detection and is capable of detecting insider threats and supply chain attacks within CIP networked environments in medium and high impact power generation sites. Additionally, the INSM has to be able to function within high impact sites without any external network connectivity.
Darktrace/OT leverages machine learning to provide actionable preventative analytics, relevant real time anomaly based threat detection, and a variety of response capabilities as a full suite protection for OT/ICS operations Purdue levels 5-0, helping security professionals accommodate for FERC order 887 requirements.
Anomaly Based Detection
Darktrace establishes baseline and normal network activity via passive traffic analysis when monitoring the CIP-networked OT system. The baseline or “pattern of life” is then used to detect anomalies within the environment including unauthorized activity, connections, devices, and software inside the CIP-networked environment via anomaly-based detection.
Darktrace’s AI technology uses unsupervised machine learning to identify anomalous activity to a high statistical level of confidence by logging network traffic via packet capture and maintaining logs and other data collected regarding network traffic inherently within the platform for 1 year.
All log data stored by Darktrace can be exported to other systems so that it can be stored longer than 1 year. If you need to retain logs for more than 1 year, Darktrace can offload the logs to retain indefinitely.
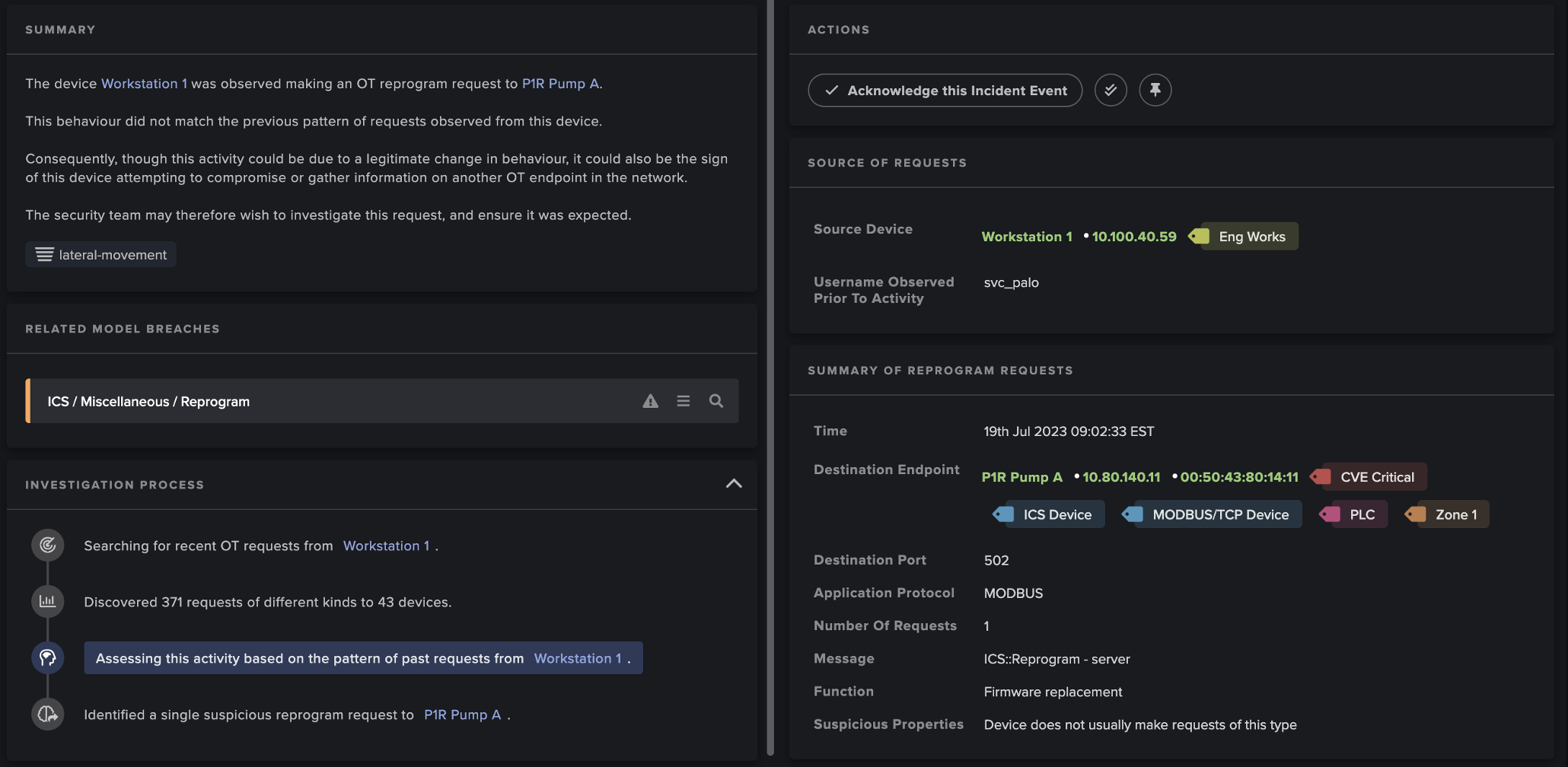
自己学習型AI
Darktrace/OT analyzes network traffic passively and learns the normal pattern of life of the these assets and their details (make, model, firmware, protocols, etc.). Darktrace/OT does not need any data or threat feeds from external sources because the AI builds an innate understanding of self without third-party support.
Darktrace is capable of detecting sophisticated novel malware-based attacks as well as supply chain attacks, insider threats, and other attacks where the adversary has established foothold or persistent legitimized access to systems and cannot be detected by rules and signatures-based detection systems.
Darktrace/OT is an intelligent decision-making engine that uses its evolving understanding of your industrial organization to prompt targeted, non-disruptive action to contain emerging attacks, actively responding to security events occurring within the security perimeter autonomously or via human confirmation using TCP/resets or Darktrace can respond at security boundaries via various integrations with network security tools including firewalls and OT zero trust solutions.
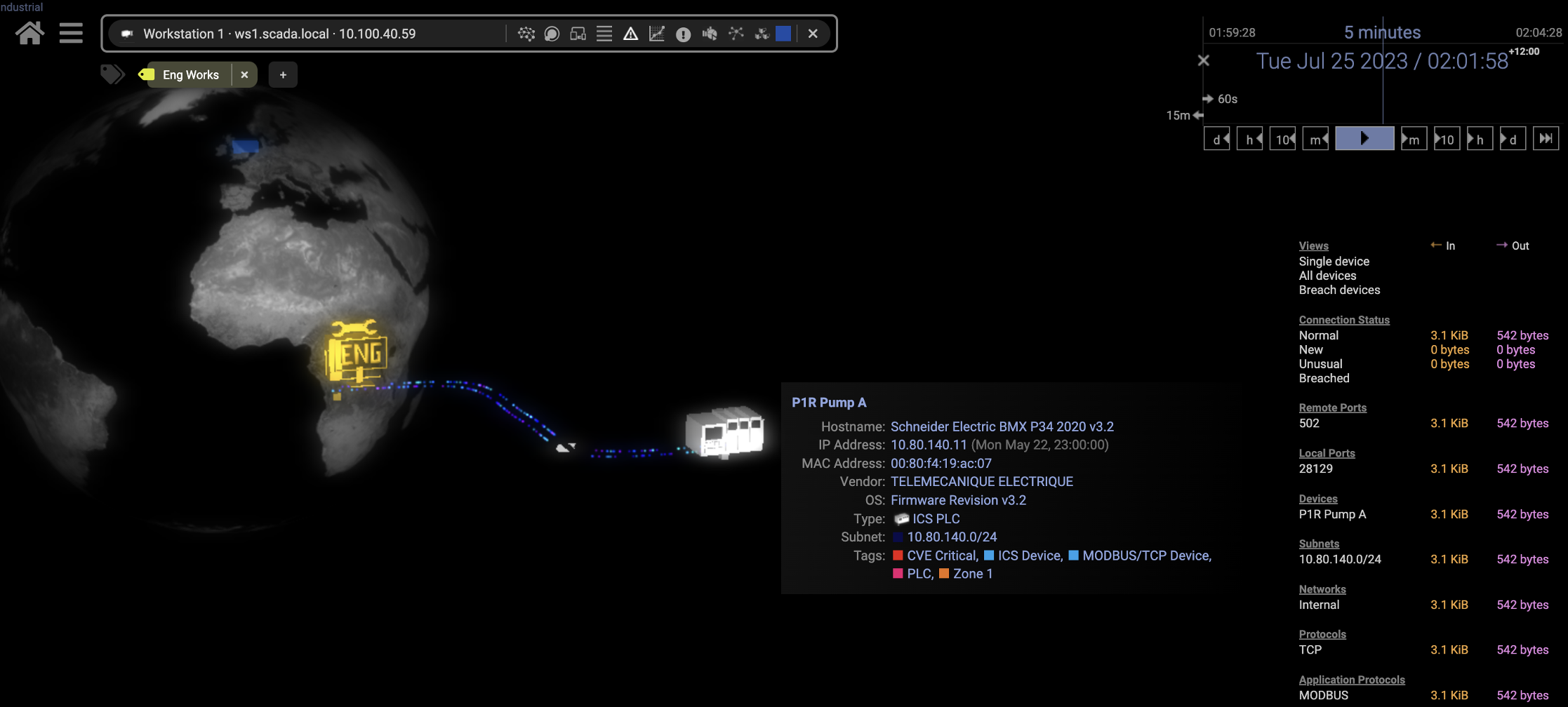
Deploys in Isolation Without External Connectivity
Darktrace/OT can deploy passively without the need for any external network connectivity into any low, medium, or high impact power generation facilities and maintain 100 percent integrity of the existing segmentation including fully air gapped environments.
Once Darktrace/OT is deployed, Darktrace immediately begins monitoring, learning, and analyzing the raw OT network traffic (east/west and north/south) within the CIP-networked environment creating a live data flow topology and baseline of network connectivity.
Because all data-processing and analytics are performed locally on the Darktrace appliance, there is no requirement for Darktrace to have a connection out to the internet. As a result, Darktrace/OT provides visibility and threat detection to air-gapped or highly segmented networks without jeopardizing their integrity. If a human or machine displays even the most nuanced forms of threatening behavior, the solution can illuminate this in real time.
Attack Case Study: Insider Threat
In the real-world example below, Darktrace/OT detected a subtle deviation from normal behavior when a reprogram command was sent by an engineering workstation to a PLC controlling a pump, an action an insider threat with legitimized access to OT systems would take to alter the physical process without any malware involved. In this instance, AI Analyst, Darktrace’s investigation tool that triages events to reveal the full security incident, detected the event as unusual based on multiple metrics including the source of the command, the destination device, the time of the activity, and the command itself.
As a result, AI Analyst created a complete security incident, with a natural language summary, the technical details of the activity, and an investigation process explaining how it came to its conclusion. By leveraging Explainable AI, a security team can quickly triage and escalate Darktrace incidents in real time before it becomes disruptive, and even when performed by a trusted insider.
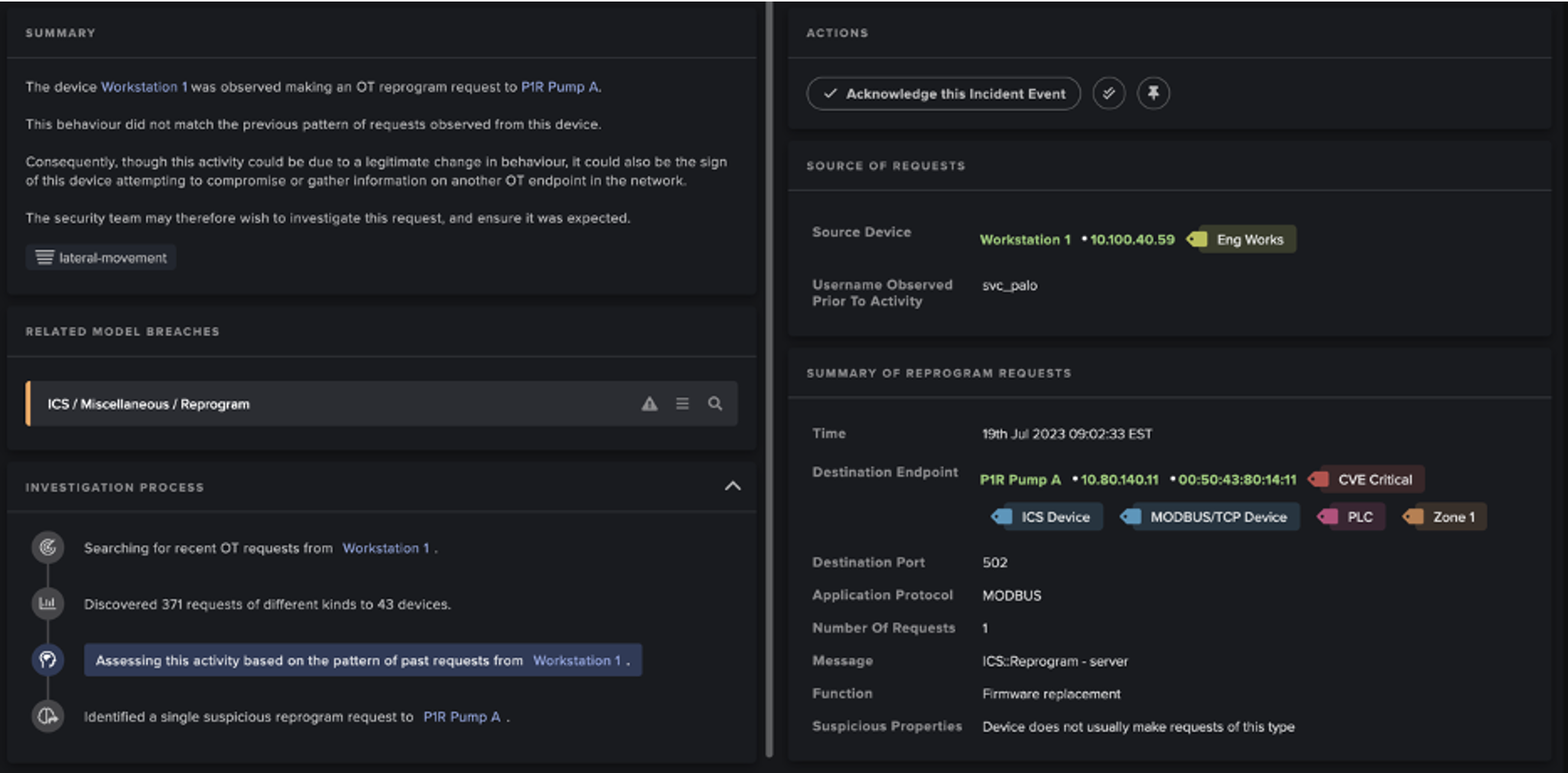
Credit to Daniel Simonds and Oakley Cox for their contribution to this blog.