攻撃者によって侵害された場合、最も被害を受けるであろう人、プロセス、テクノロジー資産は何か?
攻撃経路モデリングは、最も重要な機密情報につながるすべての道の詳細なマップを提供し、可能性と潜在的な影響の順に優先順位を付けます。CISOは、セキュリティスタックを補完するために、この種のソリューションにますます注目しています。このソリューションは、組織の構造に特有のリスクや、悪用されると致命的となるデバイスやユーザー間の予期せぬ関係の可能性を明らかにするためです。
Darktraceの攻撃経路モデリングソリューションの特徴は何ですか?
- データソースは多様で、デジタルエステート全体からの情報が考慮される
- モデリングはリアルタイムで、継続的に再評価される
- 専門的な技術的知識がなくても活用できるアウトプット
- 脆弱性の優先順位付けのためのスタンドアロン製品として活用
- サイバーAIループの構成要素として、このソリューションは、DETECTおよびRESPOND (例:重要資産へのタグ付けによる検知)にフィードバックすることで即時価値を提供しますが、結果をフォローアップすることで長期的なシステム改善も実現します。
攻撃者のように考える
2023年、CISOは単なる保険やチェックボックスのコンプライアンスにとどまらず、アンダーライターが特定の種類のサイバー攻撃に対する除外規定を設けるようになります。運用保証を強化するのではなく、保護ボックスをチェックするコンプライアンスの限界がより明確になるにつれ、その立場を変えていくでしょう。彼らは、予算が削減される中でROIを最大化するために、よりプロアクティブなサイバーセキュリティ対策を選択し、サイバーレジリエンスを継続的に改善し、サイバーリスク低減を実証するツールや機能への投資にシフトするよう、チームに働きかけるでしょう。
レッドチームは、労力とリソースを最も即座に適用すべき場所についての洞察を提供することができますが、演習自体はコストがかかり、網羅的でなく、実行頻度も低いことが多いのです。
ハッカーは、システムの脆弱性を突いて攻撃するための経路、できれば最も抵抗の少ない経路を常に探し求めています。攻撃経路のモデル化により、セキュリティチームは攻撃者の視点から自分たちの環境を見ることができます。これにより、攻撃経路を段階的に排除し、攻撃者が壁を突破する際の選択肢を減らすことができます。
攻撃経路モデリングに関する詳細
攻撃経路とは、攻撃者がシステムの弱点を突くために取る経路を視覚的に表現したものです。攻撃経路は、脅威者が組織への入り口(攻撃対象領域)から貴重な資産にアクセスするまでの一連のステップ(攻撃ベクトル)を強調するものです。
通常、攻撃者が最も欲しいデータまでまっすぐに大通りを行くことは稀です。攻撃者は、いくつかの抜け道や予期せぬ関係、セキュリティスタックの死角を利用して、機密資産への道を切り開く可能性が高いのです。攻撃経路のモデリングは、このような侵入経路を形成するために必要な攻撃ベクトルを明らかにするのに役立ちます。
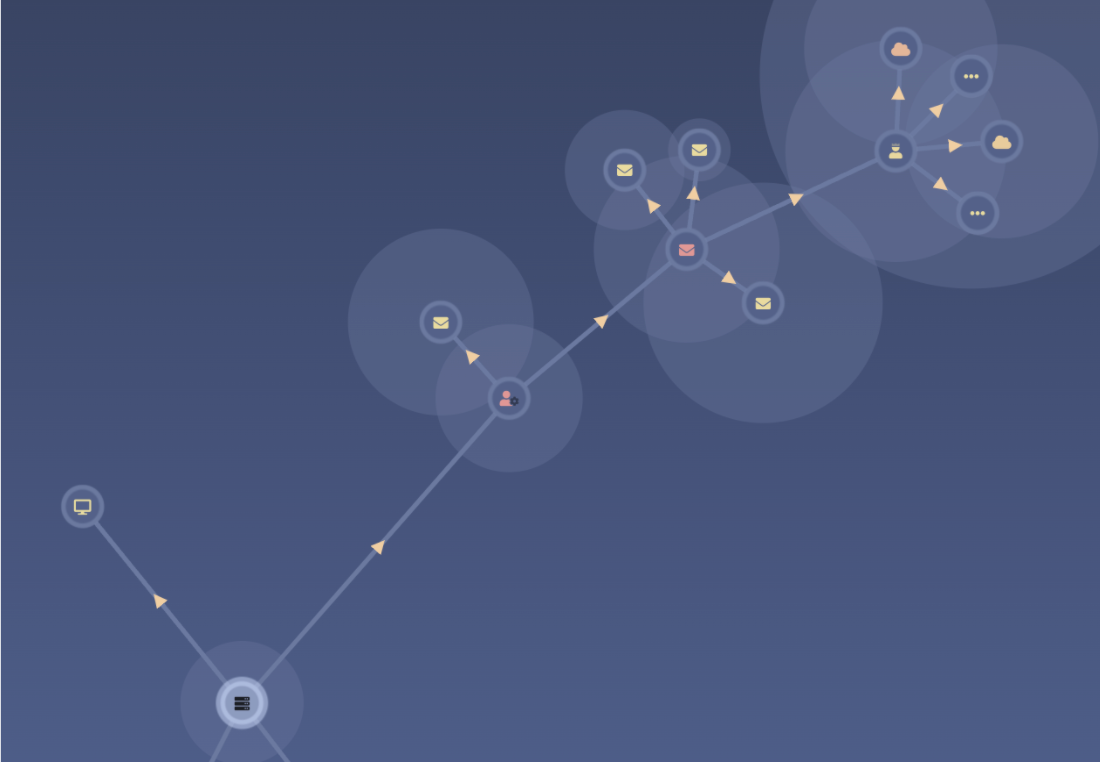
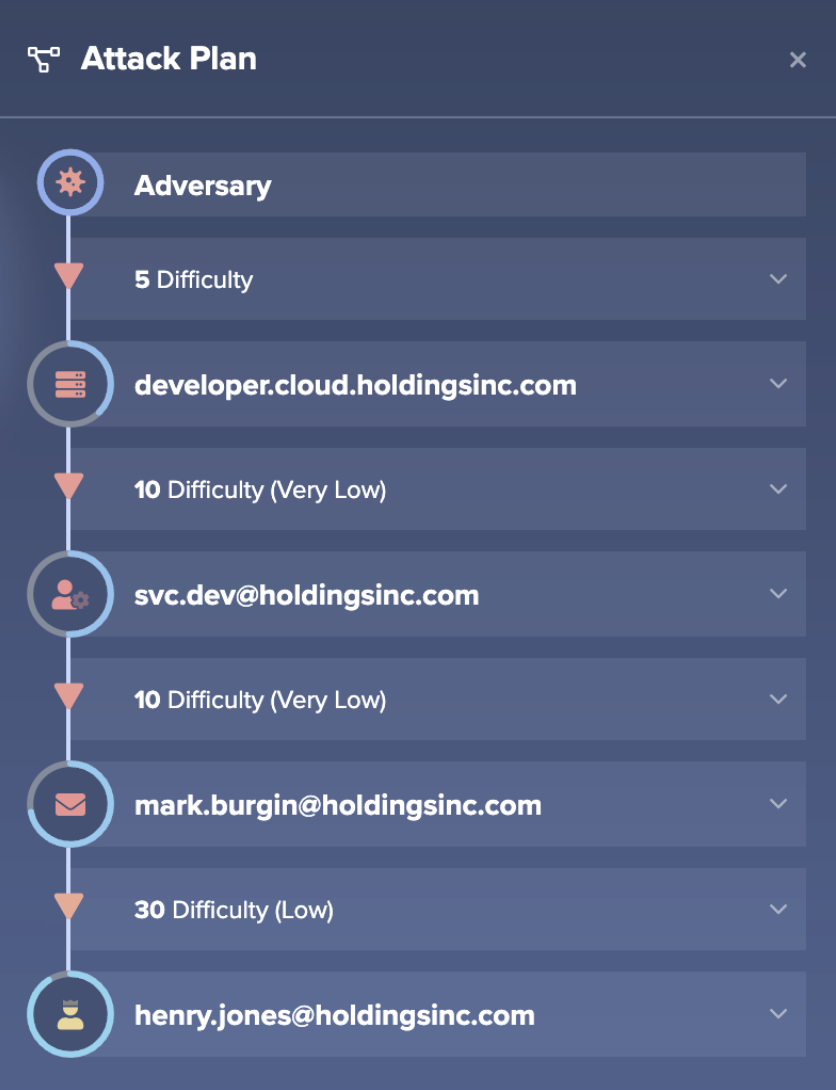
攻撃経路をモデル化する方法
Darktraceは、独自の自己学習型AIで関係性をモデル化し、グラフ理論を取り入れることで、ユーザー、ドキュメント、これらの関係性の重要性を理解しています。
Darktrace PREVENTの攻撃経路モデリングコンポーネントは、ターゲットノード(ユーザー、アカウント、デバイス)を特定し、これらのターゲットノードへの最短経路を計算し、この攻撃経路の可能性とターゲット資産が侵害された場合の被害に応じて結果に重みを付けます。これは、攻撃者が攻撃を計画する際に行うことと全く同じですが、攻撃者よりも多くの情報にアクセスできるDarktrace PREVENTのAIエンジンに大きなアドバンテージがあると言えます。このとき初めて、防御側が攻撃側に対して優位に立つことができるのです。
サイロ化した取り組みの回避
ガートナーによると、75%の組織がセキュリティツールの統合を検討しています。これは、主にコスト的な理由ではなく、サイバーリスクの低減を促進するためです。これらの投資から最大限の利益を得るためには、セキュリティへの取り組みが、サイロ化した取り組みではなく、より広範なセキュリティエコシステムの一部であることを確認することが重要です。Darktraceの攻撃経路モデリングソリューションは、Darktrace PREVENT のエンドツーエンド(E2E)サービスのコンポーネントとして提供されています。
Darktrace PREVENT は、Darktrace のDETECT およびRESPOND と統合し、攻撃経路を排除する時間がない場合でも、組織のセキュリティ体制が強化されるようにします。
防御の優位性は重要であり、攻撃経路モデリングは、セキュリティチームが優位性を取り戻すための1つの方法です。攻撃経路モデリングは、セキュリティチームが優位性を取り戻すための1つの方法です。
しかし、攻撃経路モデリングは客観的なものであり、これらのモデルを作成するさまざまな方法を評価する際に考慮すべきいくつかの重要な疑問があります。
私の攻撃経路マップを構築する際、すべての関連データを考慮しているか?
マーケティング担当の重役の一人が、開発チームの誰かと親しい友人関係にある場合を考えてみましょう。このような場合、どのように攻撃経路をモデル化すればよいのでしょうか。攻撃経路はデジタル資産全体を包含するため、攻撃経路のモデリングソリューションは、内部および外部のさまざまな部分からの情報を考慮する必要があります。これには、Eメール環境、ネットワーク、エンドポイント、SaaSとクラウド、Active Directory、脆弱性スキャナなどからのデータが含まれることがあります。
全体的な攻撃経路を把握するためには、データのクロス分析が唯一の方法です。
攻撃経路の最新のルートを理解しているのだろうか?
ユーザー、デバイス、その他の機密資産の関係は日々変化しており、これは攻撃経路が日々変化していることを意味します。セキュリティ担当者が組織のリスク状況を最新の状態で把握したいのであれば、使用する手法やソリューションが継続的かつリアルタイムに理解を更新していることを確認することが重要です。
セキュリティ体制を向上させるために、どのような攻撃経路から手をつければよいのか、どうすればよいのか?
一つは攻撃経路の総和をマップ化すること、もう一つは攻撃経路に優先順位をつけることです。攻撃経路のモデリングはマップを提供しますが、その上にリスク評価(以下で詳しく説明します)のレイヤーを追加することで、優先順位付けを行うことができます。そこで、グラフ理論が非常に有効であり、強化すべきチョークポイントを特定することができます。
この出力から実用的な洞察が得られるか?
このソリューションの主な目的は、単にサイバーリスクの状況を評価することではなく、セキュリティ対策を正しい方向に導くことにあります。そのためには、サイバー技術の専門家ではないチームメンバーも利用できるような出力が必要です。利用可能な洞察と緩和のためのアドバイスによって参入障壁を低くすることが、組織のセキュリティ体制をうまく改善する鍵になります。
攻撃経路の優先順位付けのためのリスクアセスメント
Darktraceの攻撃経路モデリング(APM)は、サイバー攻撃の経路を評価するリスクベースのアプローチで、攻撃者の立場で考え、最も抵抗の少ない経路を探り当てるものです。この場合の「リスク」は、2つの要因の積として定義されます。「確率」と「影響力」です。この情報を使って、考えられる攻撃経路を以下のリスクマトリックスに分類することで、DarktraceのAPMは攻撃経路に優先順位を付け、セキュリティチームの労力を組織にとって最も関連性の高いリスクの制御に費やすことができるようにすることができます。
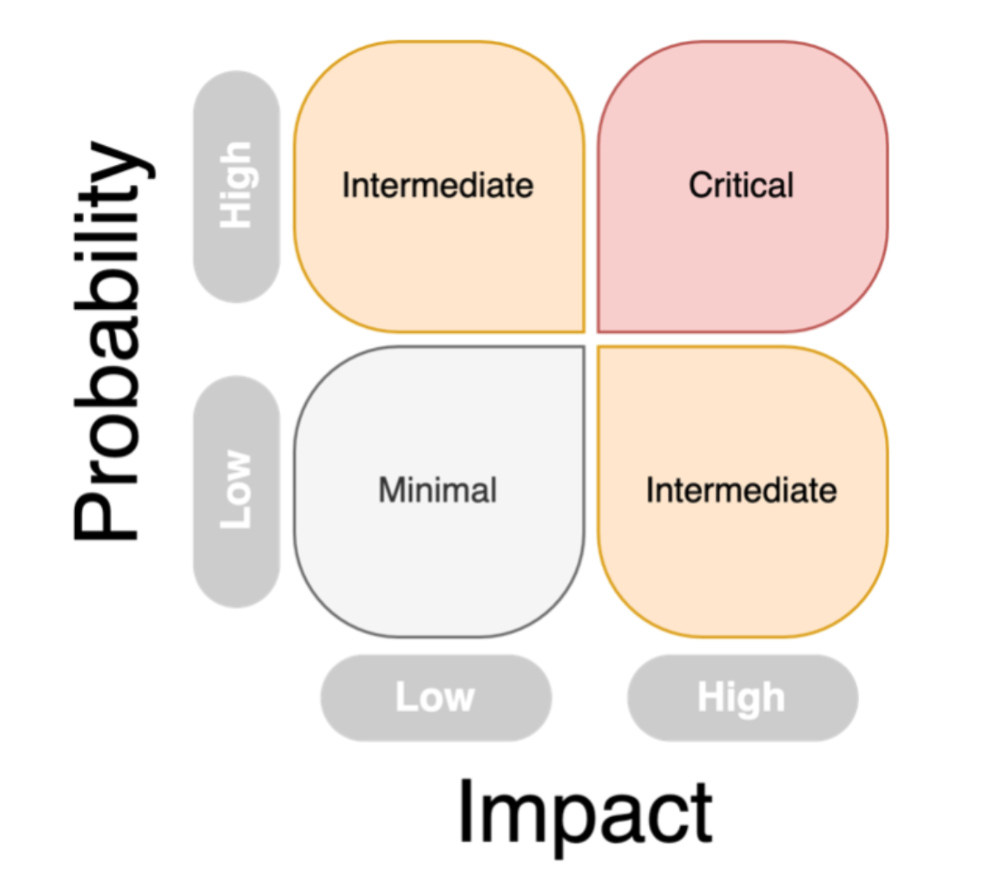
A: 確率の定義
確率には2つのタイプがあります。
攻撃者が組織に侵入するために、ある特定のドアを選択する可能性(攻撃対象地域の資産のうち、インターネットに面したサーバー、受信トレイ、SaaSおよびクラウドアカウントなどが考えられる)
ある特定のノード(デバイスまたはユーザーアカウントと定義される)が、ラテラルムーブメントによって次に侵害される可能性

B: インパクトの定義
インパクトとは、ある資産が侵害され使用できなくなった場合の総合的な影響度を指します。資産(例:キーサーバー)の場合、この資産が停止した場合の混乱が大きければ大きいほど、インパクトのスコアが高くなります。特定の文書を考える場合、アクセス制限やアクセスするユーザーの機密性スコアなどが影響度の推定に使われる変数の一部です。
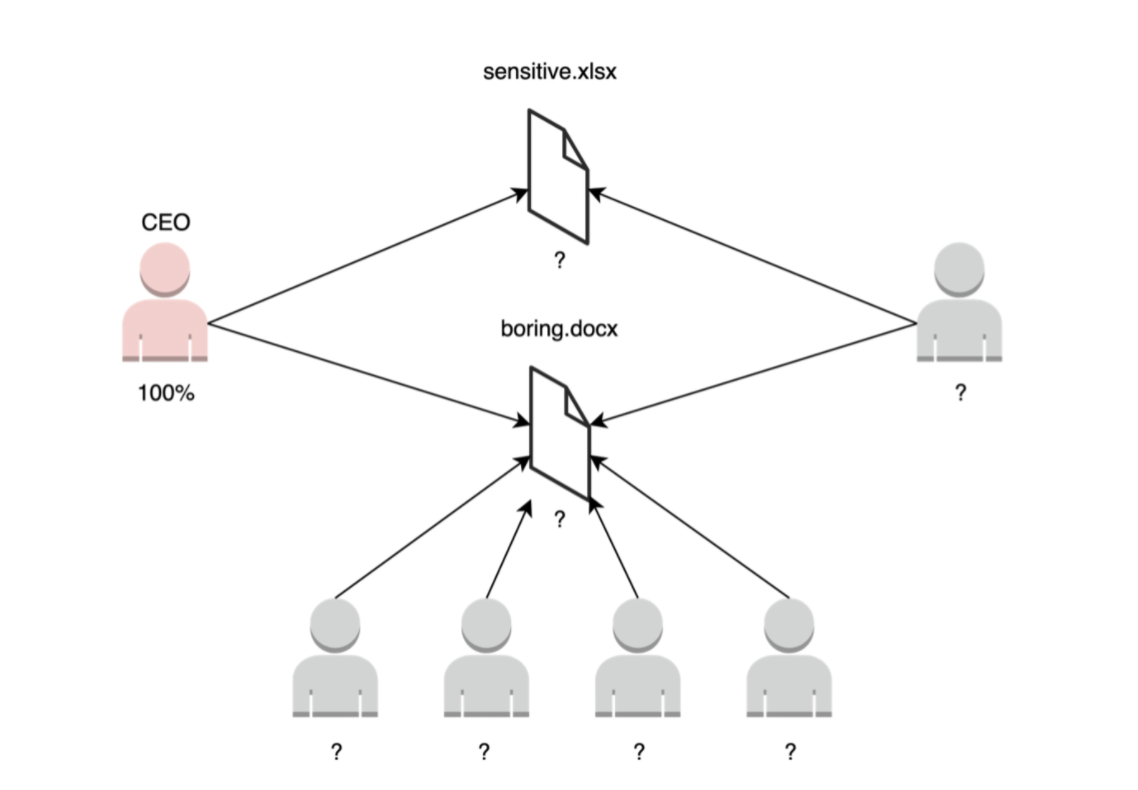
どちらの変数も、人間の入力を必要とせず、AIによって自律的に計算されます。もちろん、セキュリティチームはAIによる組織への理解をビジネス上の専門知識で補強することができます(たとえば、機密性の高いデバイスに追加でタグを付けるなど)。
Darktrace Attack Path Modeling モジュールを構成する他のコンポーネントと同様に、キーサーバーや機密文書を特定するためにどのように影響が伝播されるかについてのより詳細な説明は、このホワイトペーパーに記載されています。